In the event you’re an AI chief, you may really feel such as you’re caught between a rock and a tough place currently.
It’s important to ship worth from generative AI (GenAI) to maintain the board joyful and keep forward of the competitors. However you additionally have to remain on prime of the rising chaos, as new instruments and ecosystems arrive available on the market.
You additionally need to juggle new GenAI tasks, use instances, and enthusiastic customers throughout the group. Oh, and information safety. Your management doesn’t wish to be the subsequent cautionary story of fine AI gone unhealthy.
In the event you’re being requested to show ROI for GenAI nevertheless it feels extra such as you’re enjoying Whack-a-Mole, you’re not alone.
In keeping with Deloitte, proving AI’s enterprise worth is the highest problem for AI leaders. Corporations throughout the globe are struggling to maneuver previous prototyping to manufacturing. So, right here’s methods to get it finished — and what you must be careful for.
6 Roadblocks (and Options) to Realizing Enterprise Worth from GenAI
Roadblock #1. You Set Your self Up For Vendor Lock-In
GenAI is shifting loopy quick. New improvements — LLMs, vector databases, embedding fashions — are being created every day. So getting locked into a particular vendor proper now doesn’t simply threat your ROI a yr from now. It might actually maintain you again subsequent week.
Let’s say you’re all in on one LLM supplier proper now. What if prices rise and also you wish to swap to a brand new supplier or use totally different LLMs relying in your particular use instances? In the event you’re locked in, getting out might eat any price financial savings that you simply’ve generated along with your AI initiatives — after which some.
Resolution: Select a Versatile, Versatile Platform
Prevention is the very best treatment. To maximise your freedom and flexibility, select options that make it simple so that you can transfer your whole AI lifecycle, pipeline, information, vector databases, embedding fashions, and extra – from one supplier to a different.
For example, DataRobot offers you full management over your AI technique — now, and sooner or later. Our open AI platform allows you to preserve complete flexibility, so you need to use any LLM, vector database, or embedding mannequin – and swap out underlying parts as your wants change or the market evolves, with out breaking manufacturing. We even give our prospects the entry to experiment with frequent LLMs, too.
Roadblock #2. Off-the-Grid Generative AI Creates Chaos
In the event you thought predictive AI was difficult to manage, strive GenAI on for measurement. Your information science group doubtless acts as a gatekeeper for predictive AI, however anybody can dabble with GenAI — and they’re going to. The place your organization might need 15 to 50 predictive fashions, at scale, you might nicely have 200+ generative AI fashions everywhere in the group at any given time.
Worse, you won’t even learn about a few of them. “Off-the-grid” GenAI tasks have a tendency to flee management purview and expose your group to vital threat.
Whereas this enthusiastic use of AI could be a recipe for better enterprise worth, in reality, the other is usually true. And not using a unifying technique, GenAI can create hovering prices with out delivering significant outcomes.
Resolution: Handle All of Your AI Property in a Unified Platform
Combat again in opposition to this AI sprawl by getting all of your AI artifacts housed in a single, easy-to-manage platform, no matter who made them or the place they had been constructed. Create a single supply of fact and system of file in your AI property — the way in which you do, for example, in your buyer information.
After getting your AI property in the identical place, then you definately’ll want to use an LLMOps mentality:
- Create standardized governance and safety insurance policies that can apply to each GenAI mannequin.
- Set up a course of for monitoring key metrics about fashions and intervening when essential.
- Construct suggestions loops to harness consumer suggestions and repeatedly enhance your GenAI functions.
DataRobot does this all for you. With our AI Registry, you possibly can arrange, deploy, and handle your whole AI property in the identical location – generative and predictive, no matter the place they had been constructed. Consider it as a single supply of file in your whole AI panorama – what Salesforce did in your buyer interactions, however for AI.
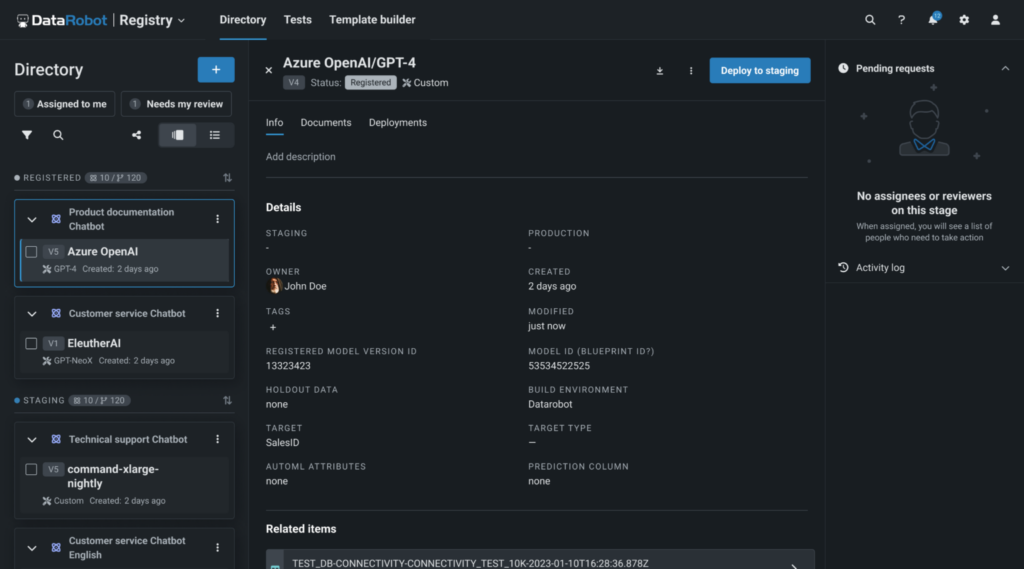
Roadblock #3. GenAI and Predictive AI Initiatives Aren’t Beneath the Similar Roof
In the event you’re not integrating your generative and predictive AI fashions, you’re lacking out. The facility of those two applied sciences put collectively is a large worth driver, and companies that efficiently unite them will be capable of understand and show ROI extra effectively.
Listed below are just some examples of what you might be doing for those who mixed your AI artifacts in a single unified system:
- Create a GenAI-based chatbot in Slack in order that anybody within the group can question predictive analytics fashions with pure language (Assume, “Are you able to inform me how doubtless this buyer is to churn?”). By combining the 2 sorts of AI know-how, you floor your predictive analytics, carry them into the every day workflow, and make them way more useful and accessible to the enterprise.
- Use predictive fashions to manage the way in which customers work together with generative AI functions and scale back threat publicity. For example, a predictive mannequin might cease your GenAI software from responding if a consumer offers it a immediate that has a excessive likelihood of returning an error or it might catch if somebody’s utilizing the appliance in a method it wasn’t supposed.
- Arrange a predictive AI mannequin to tell your GenAI responses, and create highly effective predictive apps that anybody can use. For instance, your non-tech workers might ask pure language queries about gross sales forecasts for subsequent yr’s housing costs, and have a predictive analytics mannequin feeding in correct information.
- Set off GenAI actions from predictive mannequin outcomes. For example, in case your predictive mannequin predicts a buyer is prone to churn, you might set it as much as set off your GenAI software to draft an e mail that can go to that buyer, or a name script in your gross sales rep to comply with throughout their subsequent outreach to save lots of the account.
Nevertheless, for a lot of firms, this degree of enterprise worth from AI is unattainable as a result of they’ve predictive and generative AI fashions siloed in numerous platforms.
Resolution: Mix your GenAI and Predictive Fashions
With a system like DataRobot, you possibly can carry all of your GenAI and predictive AI fashions into one central location, so you possibly can create distinctive AI functions that mix each applied sciences.
Not solely that, however from contained in the platform, you possibly can set and observe your business-critical metrics and monitor the ROI of every deployment to make sure their worth, even for fashions working exterior of the DataRobot AI Platform.
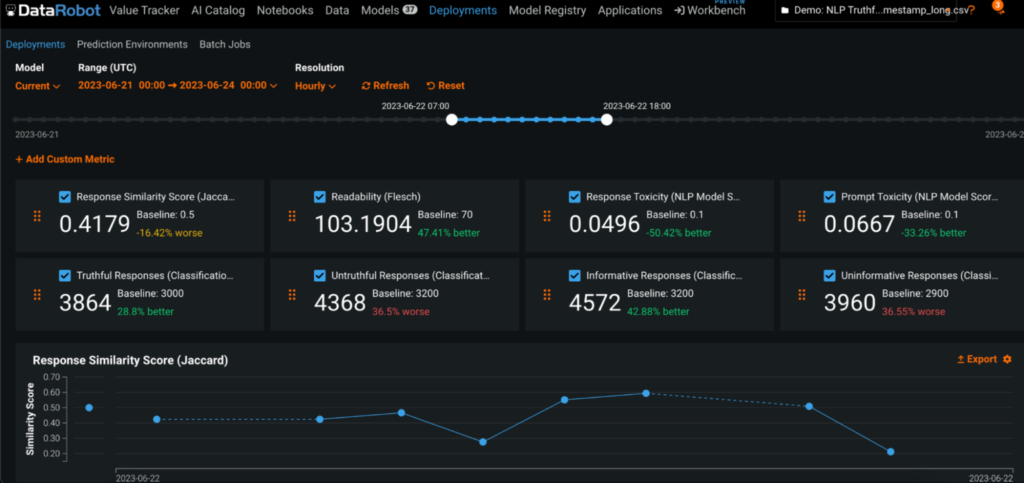
Roadblock #4. You Unknowingly Compromise on Governance
For a lot of companies, the first objective of GenAI is to save lots of time — whether or not that’s lowering the hours spent on buyer queries with a chatbot or creating automated summaries of group conferences.
Nevertheless, this emphasis on pace usually results in corner-cutting on governance and monitoring. That doesn’t simply set you up for reputational threat or future prices (when your model takes a serious hit as the results of a knowledge leak, for example.) It additionally means that you would be able to’t measure the price of or optimize the worth you’re getting out of your AI fashions proper now.
Resolution: Undertake a Resolution to Shield Your Knowledge and Uphold a Sturdy Governance Framework
To unravel this concern, you’ll must implement a confirmed AI governance software ASAP to observe and management your generative and predictive AI property.
A strong AI governance answer and framework ought to embody:
- Clear roles, so each group member concerned in AI manufacturing is aware of who’s answerable for what
- Entry management, to restrict information entry and permissions for modifications to fashions in manufacturing on the particular person or function degree and shield your organization’s information
- Change and audit logs, to make sure authorized and regulatory compliance and keep away from fines
- Mannequin documentation, so you possibly can present that your fashions work and are match for objective
- A mannequin stock to manipulate, handle, and monitor your AI property, no matter deployment or origin
Present finest follow: Discover an AI governance answer that may forestall information and data leaks by extending LLMs with firm information.
The DataRobot platform consists of these safeguards built-in, and the vector database builder allows you to create particular vector databases for various use instances to raised management worker entry and ensure the responses are tremendous related for every use case, all with out leaking confidential data.
Roadblock #5. It’s Powerful To Preserve AI Fashions Over Time
Lack of upkeep is among the largest impediments to seeing enterprise outcomes from GenAI, in keeping with the identical Deloitte report talked about earlier. With out glorious maintenance, there’s no method to be assured that your fashions are performing as supposed or delivering correct responses that’ll assist customers make sound data-backed enterprise selections.
Briefly, constructing cool generative functions is a good start line — however for those who don’t have a centralized workflow for monitoring metrics or repeatedly bettering primarily based on utilization information or vector database high quality, you’ll do one in all two issues:
- Spend a ton of time managing that infrastructure.
- Let your GenAI fashions decay over time.
Neither of these choices is sustainable (or safe) long-term. Failing to protect in opposition to malicious exercise or misuse of GenAI options will restrict the longer term worth of your AI investments virtually instantaneously.
Resolution: Make It Simple To Monitor Your AI Fashions
To be useful, GenAI wants guardrails and regular monitoring. You want the AI instruments obtainable in an effort to observe:
- Worker and customer-generated prompts and queries over time to make sure your vector database is full and updated
- Whether or not your present LLM is (nonetheless) the very best answer in your AI functions
- Your GenAI prices to be sure you’re nonetheless seeing a constructive ROI
- When your fashions want retraining to remain related
DataRobot may give you that degree of management. It brings all of your generative and predictive AI functions and fashions into the identical safe registry, and allows you to:
- Arrange customized efficiency metrics related to particular use instances
- Perceive normal metrics like service well being, information drift, and accuracy statistics
- Schedule monitoring jobs
- Set customized guidelines, notifications, and retraining settings. In the event you make it simple in your group to take care of your AI, you received’t begin neglecting upkeep over time.
Roadblock #6. The Prices are Too Excessive – or Too Onerous to Observe
Generative AI can include some severe sticker shock. Naturally, enterprise leaders really feel reluctant to roll it out at a enough scale to see significant outcomes or to spend closely with out recouping a lot by way of enterprise worth.
Retaining GenAI prices underneath management is a large problem, particularly for those who don’t have actual oversight over who’s utilizing your AI functions and why they’re utilizing them.
Resolution: Observe Your GenAI Prices and Optimize for ROI
You want know-how that allows you to monitor prices and utilization for every AI deployment. With DataRobot, you possibly can observe the whole lot from the price of an error to toxicity scores in your LLMs to your general LLM prices. You possibly can select between LLMs relying in your software and optimize for cost-effectiveness.
That method, you’re by no means left questioning for those who’re losing cash with GenAI — you possibly can show precisely what you’re utilizing AI for and the enterprise worth you’re getting from every software.
Ship Measurable AI Worth with DataRobot
Proving enterprise worth from GenAI shouldn’t be an unattainable process with the precise know-how in place. A latest financial evaluation by the Enterprise Technique Group discovered that DataRobot can present price financial savings of 75% to 80% in comparison with utilizing current sources, providing you with a 3.5x to 4.6x anticipated return on funding and accelerating time to preliminary worth from AI by as much as 83%.
DataRobot may also help you maximize the ROI out of your GenAI property and:
- Mitigate the chance of GenAI information leaks and safety breaches
- Hold prices underneath management
- Carry each single AI venture throughout the group into the identical place
- Empower you to remain versatile and keep away from vendor lock-in
- Make it simple to handle and preserve your AI fashions, no matter origin or deployment
In the event you’re prepared for GenAI that’s all worth, not all discuss, begin your free trial right now.
Concerning the creator
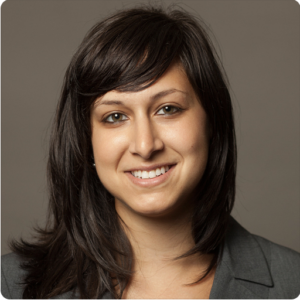
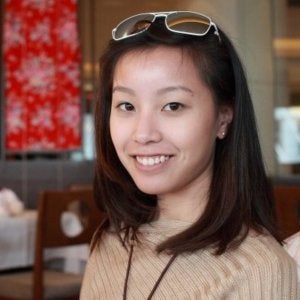
Joined DataRobot by means of the acquisition of Nutonian in 2017, the place she works on DataRobot Time Collection for accounts throughout all industries, together with retail, finance, and biotech. Jessica studied Economics and Laptop Science at Smith Faculty.